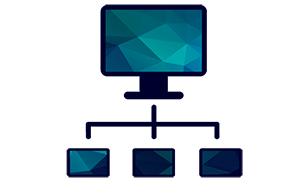
Big Data
Big Data
The recent increase in availability of large datasets from a variety of sources has opened a new dimension in predictive analytics space, enabling financial institutions to take advantage of such large source of data, along with it existing traditional data, to gain useful insights that can reap commercial, business and IT benefits to the companies and to their customers. A key PREDMINE advantage is that it frees its customers of the need to develop Big Data solutions of their own for their predictive analysis needs.
For insurance companies hoping to tap into the increased insight and productivity of Big Data – and, therefore, the increased revenue as well as the potential to reduce their cost base – they will need to ensure they have the right systems in place to cope with both structured and unstructured data.
Insurance is a data-driven industry, and insurance companies employ large numbers of analysts to understand claims data. The statistical methods used to estimate typical claim sizes are fundamentally different that those used to assess the probability of infrequent larger claims. Diverse and dynamic contexts require that empirical data be modelled per place and population to accurately measure change and identify trends. Such models are crucial to all business decisions pertaining to expected profits, premium loadings, reserves necessary to ensure (with high probability) profitability, and the impact of reinsurance and deductibles.
Web Mining
One of the largest challenges insurers will face in 2014 and beyond is capturing and interpreting data from a growing number of structured and unstructured sources. Insurers that apply advanced analytical techniques to harness the power of big data will be better able to understand their customers, tailor products to meet their needs, and enhance the overall customer experience.
Where sufficient loss or claim experience to produce reliable trend indications is not available, the actuary might supplement or supplant the available experience with external data through text mining techniques. Insurance trade associations, statistical bureaus, police reports, information through Internet sources and the Government produce insurance related and general economic data regularly.
If past is any guide for predicting future events, predictive modelling is an excellent technique for insurance risk management. Predictive models are developed from past historical records of insurance policies, containing financial, demographic, psychographic, geographic information, along with properties of insured objects. From the past insurance policy information, predictive models can learn patterns of different insurance claim ratios, and can be used to predict risk levels of future insurance policies. It is important to note that statistical process requires a substantially large number of past historical records (or insurance policies) containing useful information. Useful information is something that can be a factor that differentially affects insurance claims ratios.